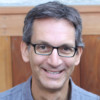
[
Karl R. Pfleger 1st degree connection 1st Longevity Investor & Philanthropist. Founder, AgingBiotech.info.
](https://www.linkedin.com/in/karl-r-pfleger/)
11h
Nice article. Will help get wider exposure to the field in the same way Bryan Johnson’s press has. But when are you going to expand to better methylation clocks than just TruMe? The field will soon need an independent leaderboard with the best clocks plus functional measures. Unlikely that this is true LEV.
5 Replies 5 Replies on Karl R. Pfleger’s comment
[
Tom WeldonView Tom Weldon’s profile Author Founder, Chairman and CEO at Ponce De Leon Health
](https://www.linkedin.com/in/ACoAACXfJoMBf_fJbhrMa__1pY2240DVOLf5zqk)
10h
Hi Karl,
Although this regression line is impressive, 6 data points would be reasonable to forecast out two more points, obviously not forever. But the story will hopefully get exposure to the industry, which is critically important, especially now. In terms of the TruMe test, it actually has been validated against one of the later Horvath clocks, and of course, “accuracy” is simply conceptual. The biological age produced by any algorithm is simply an estimation, and whether one clock gives a different answer that is 5 years higher or lower than another, is probably not the most important point. Taking multiple readings over several years is what matters. Are the data consistent, is there a trend? I have over 4 years worth of data, and the first 3.5 years of data have an upward trend, similar to the increase in my chronologic age, then the trend is pronouncedly downward. Having worked in the Medtech field most of my life, I am very familiar with what the FDA will probably require for a new endpoint to be valid for FDA approval purposes. It will require a drug like trial run until all subjects have expired to prove that a particular clock is “accurate”. No one will do such a trial, which is why I chose compounds that are not drugs.
[
Hannah WentView Hannah Went’s profile (She/Her) • 1st Director of Operations at TruDiagnostic
](https://www.linkedin.com/in/ACoAADAEU_0B5PF2DAIsXYcTqxOUwE3KnJg7GqM)
9h (edited)
Karl R. Pfleger Tom Weldon I think another important point is that we do not want these clocks to be accurate, as that would just mean they are getting closer and closer to your chronological age - the first generation clocks. Examples of the first generation clocks include the Horvath clock, Hannum clock, etc. These clocks still have great application. For example, they were first used to help refugees seek asylum (https://www.researchgate.net/publication/327420654_European_scientists_seek_‘epigenetic_clock’_to_determine_age_of_refugees) and to identify the age of DNA at crime scenes. However, there are better predictors of biological age that are more clinically relevant - second generation clocks (and third generation - DunedinPACE). The second gen clocks are trained using an underlying aging phenotype rather than just chronological age. In addition, these clocks are much more precise than the first gen clocks, which is exactly what you would want for a clinical trial. We need to know that the results are a true change of underlying biology rather than just noise from the algorithm itself. Morgan Levine’s Principal-Component Clocks (second generation) greatly help with the precision as well!
[
Karl R. PflegerView Karl R. Pfleger’s profile • 1st Longevity Investor & Philanthropist. Founder, AgingBiotech.info.
](https://www.linkedin.com/in/ACoAACXrfxEBV4Mx4ibKNRbvWtTtsCO6XMZSm74)
8h
Hannah Went When I said better I meant in terms of mortality prediction & by extension prediction of age-related diseases. PC-GrimAge is probably the best current clock for this in research settings. Elysium has it’s Index clock (which Levine helped design when she was consulting there and which does test many sites to get the same PC benefit)—would be nice to see some good direct comparison of these 2.
DunedinPACE is also supposed to be good but the rate-of-aging measure is a different kind of thing and not as useful (IMO) when used to sample sparsely & longitudinally as Tom is doing here. (I think TruDiagnostic’s DTC clock does give a point-estimate of current bio-age too, but I don’t know the details of how that works. Note: TruDiagnostic should not be confused with TruMe labs.)
[
Karl R. PflegerView Karl R. Pfleger’s profile • 1st Longevity Investor & Philanthropist. Founder, AgingBiotech.info.
](https://www.linkedin.com/in/ACoAACXrfxEBV4Mx4ibKNRbvWtTtsCO6XMZSm74)
7h
Karl R. Pfleger Hannah, perhaps you can elaborate on your point-estimate?
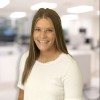
[
Hannah Went (She/Her) 1st degree connection 1st Director of Operations at TruDiagnostic
](https://www.linkedin.com/in/thehannahwent/)
6h
Karl R. Pfleger I agree!
As for the current clocks TruDiagnostic offers, all of them are principal component controlled. This was a large platform updated we did in spring of 2021.
As we realize the need for better predictors, TruDiagnostic is currently creating an algorithm (called OmicAge) with Harvard which was trained on 5,000 patients with full metabolomic data, large scale proteomic data, methylation and 75 clinical variables taken at 4 time points. We’ve spent the last 2.5 years creating it and will be releasing a preprint on it soon. In the meantime, I’m happy to privately send you the hazard ratio relationship to disease versus the other clocks. It significantly outperforms every clock including the DunedinPace!